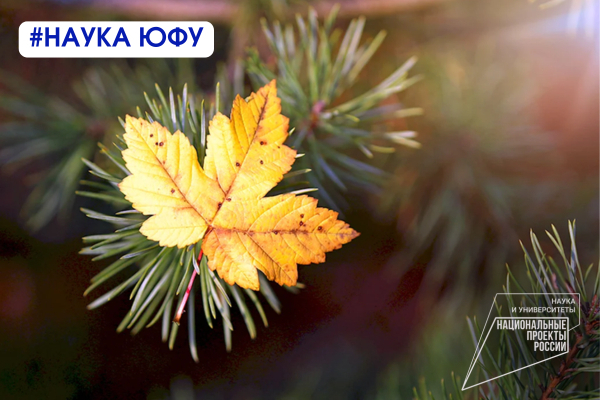
SFedU scientists have developed a new method for remote diagnosis of coniferous trees, based on hyperspectral imaging, which will help effectively determine the phases of the plant life cycle — vegetation and rest. These data are especially important for determining the degree of frost resistance of trees and can play a key role in managing forest plantations in the face of climate change.
All woody plants growing in the temperate climate of the northern hemisphere undergo a complex process of acclimatization or hardening in autumn, allowing them to endure winter with negative temperatures. The acquisition of frost resistance during this process is associated with physiological, biochemical changes that lead to dehydration of tissues and accumulation of organic antifreeze in cells, which prevents the formation of ice crystals and cell destruction. At the same time, growth inhibitors are also formed in the tissues, preventing the untimely awakening of plants. This state is called a state of rest, unlike vegetation, when there is visible growth and development of plants. No less difficult is the reverse process of transition from a state of rest to a state of vegetation – deacclimatization. To determine the resting state and assess its depth (the degree of winter hardiness of plants), there are quite complex biophysical, physiological and biochemical methods that must be used in a complex. These methods are quite accurate, but require damage to the object and do not allow an assessment to be made quickly and over large areas. If the beginning of the acclimatization process can be determined in deciduous plants during phenological observations (the acclimatization process begins immediately after leaf fall), then it is impossible to do this in evergreen plants.
Hyperspectral phenotyping is an advanced non—invasive method that uses the analysis of the spectrum of light reflected by plants in different ranges — from ultraviolet to infrared. Scientists from the Southern Federal University, using the Cubert UHD-185 hyperspectral camera for drones, sensitive to the smallest color change, took pictures of several species of coniferous plants from the collection of the Southern Federal University Botanical Garden, including western thuja, oriental flatwood, scots pine and folded thuja, during an annual cycle. The data was then analyzed using machine learning methods, which made it possible to diagnose with high accuracy whether the plants are in a state of vegetation or dormancy. It is noteworthy that, depending on the resolution of the camera used, the condition of both individual trees and the forest can be assessed in this way.
The results of the study showed that key indicators, such as the content of chlorophyll and carotenoids, play an important role in determining the degree of frost resistance of plants. This technique allows you to effectively monitor the state of forests in large areas without the need to damage trees.
"We conducted a search study to determine the possibilities of hyperspectral phenotyping methods for diagnosing dormant and vegetative conditions in evergreen conifers. During a laboratory experiment using machine learning methods and a detailed annual time series of spectral characteristics of coniferous shoots, it was possible to diagnose the conditions of "vegetation" and "rest" with high accuracy. The vegetation indices that are the most informative for describing the annual cycle of coniferous plants development have been determined. The results have been obtained substantiating the possibility of determining the degree of frost resistance of various species of coniferous plants by their spectral characteristics," said Pavel Dmitriev, head of the project supported by the RNF grant, Candidate of Biological Sciences, senior researcher at the SFedU Botanical Garden.
As the researchers explained, a pre-trained machine learning algorithm correlated the color saturation of the images with the condition of the plants. The algorithm assigned the greatest weight to the part of the spectral data that displayed the amount of pigments (for example, chlorophyll and carotenoids) in plant shoots. This characteristic was important in the analysis, since insufficient pigmentation often indicates a disease.
Figure. Seasonal dynamics of vegetation indices PRI_norm (a), PRI (b), CCI (c), PRI/CI2 (d), DPI (e) and NDVI (f) in comparison with the dynamics of daily temperature.
The most effective way (with an accuracy of 97.7%) was to determine the condition of the flatworm (Platycladus orientalis), while for other species the accuracy ranged from 92.3% to 92.9%. This is due to the fact that the leaves of the flatworm contain many pigments — chlorophyll and carotenoids, to which the proposed system reacts well.
The developed approach will not only accelerate the diagnosis of the condition of coniferous plants, but also help in solving forestry issues, especially in the context of climate change and its impact on tree health.
The authors note that in the future they plan to develop methods for remote diagnosis of vegetation, rest, acclimatization and deacclimatization conditions.
The research results have been published in prestigious scientific journals such as Horticulturae and Remote Sensing Applications: Society and Environment. These works are supported by the grant of the Russian Science Foundation No. 23-24-00390.
Short link to this page sfedu.ru/news/76259